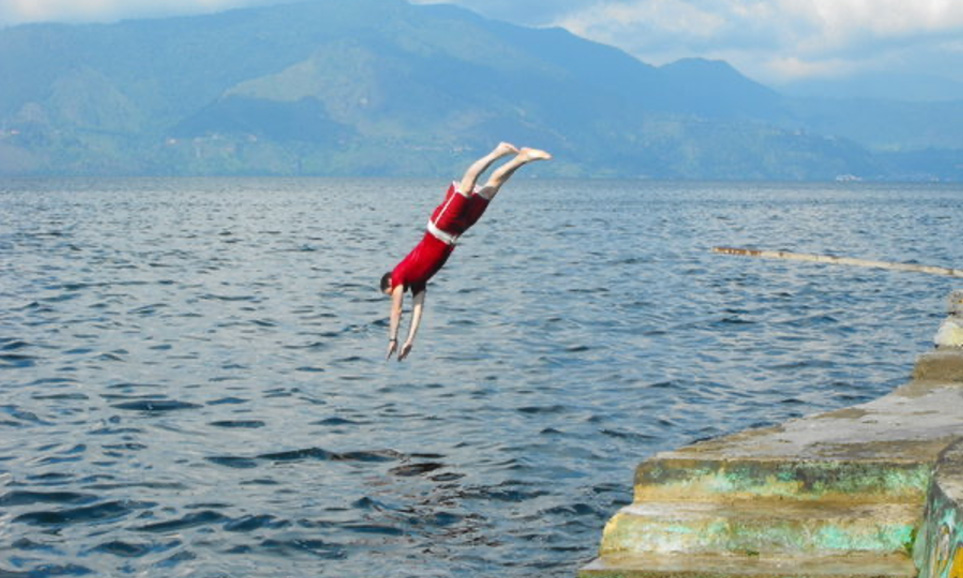
How to interpret and visualise data before diving in.
In this blog Matthew Hopkinson illustrates why understanding how to use data and software to solve problems is not as common and effective as it might appear. He uses three case studies of data interpretation from innovative companies in this space.
Covid19 has significantly increased the awareness of the importance of data when making informed decisions and evidencing them to the communities affected. Covid19 has also flagged up inconsistencies in data capture, data tagging and data currency when measuring key indicators at local, regional, national and international levels. The complexity of analysis increases when one analyses the relationship between multiple data such as in The Economist charts below.

Understanding the data that you are analysing, deciding how you analyse it and answering the ‘SO WHAT’ has become more complicated, fast flowing and challenging than ever before. Understanding the question you are trying to answer is a fundamental first stage of this exercise that many seem to ignore and just dive into the ‘data lake’!

I am fortunate and privileged to be able to work with companies who have best in class expertise in data aggregation, data integrity, data visualisation and most importantly a relentless focus on helping their clients challenge themselves to answer the questions they need answering or solving the problems that need solving.
In 2020 we have the computing power, we have the data (good and bad, structured and unstructured), we have the data science skills but often the component that is lacking is the data story telling. All too often we get overly complex and technical responses from highly technical individuals that we find difficult to relate to the practical issue we are trying to solve. To many this is intimidating so challenging or qualifying what is being presented is out of the question. As humans we have grown up being told stories and telling stories through data is no different to telling stories through words.

“As humans we have grown up being told stories and telling stories through data is no different to telling stories through words.”
For over ten years I have banged the ’data as the evidence base’ drum for repurposing our towns and cities but to little effect I am sorry to say. People and the places we populate are a critical part of the society that we live in. In any other industry or sector you would be guided by data being presented to you from driving a car, flying a plane, cycling via apps such as Strava and analysing as well as trading stocks and shares to name but a few. When it comes to our towns no such resource exists even at an open data level.
If we are to understand what is happening in our towns and communities we need a dynamic evidence base to interrogate, in order to answer our questions and solve the many challenges that exist now and in the future. This evidence base needs to cover economic, social and environmental data. The data will be derived from both within a place through community engagement and from data about that place (open and commercial datasets).

“If we are to understand what is happening in our towns and communities we need a dynamic evidence base to interrogate …”
To illustrate that this is possible I am going to show you some of the innovative ways that the companies I work with deliver this today.
Covid19 – Places and People
Recently, in response to the demand to understand the impacts of Covid19, up and down England. the team at Emu Analytics have leveraged their data visualisation and analytics platform to present a pre’ and ‘post’ Covid19 (easing of Lockdown!!) along with the change between the two. They have collated a broad range of relevant open data assets to understand the socio-economic impact of COVID-19, and visualised these through their platform, Location Insights Explorer, to give government bodies an accessible tool to identify which parts of their communities are key action zones for early intervention. Local Authorities (.gov.uk email addresses) can access this for free for the rest of this year.
The key attributes here is that it enables you to easily navigate to your town, there is clarity as to what the visualised data contains and there is a colour code value indicator. The broad range of datasets are sorted into key subject areas;
*I have centred the maps on Leicester in light of it being the first new Lockdown city and the closes city to where I live!
Economic vulnerability.
This includes the Index of Multiple Deprivation, Housing Benefit and Universal Credit counts, Median House Price, Household Income, Output Area Classification, Consumer Vulnerability, Mortgage Lending and data from the British Red Cross COVID vulnerability index.

Employment Vulnerability
The data in this section includes Employment Deprivation (IMD 2019), JSA, Income Support, Employment levels, Education levels, percentage of the population aged 25-34, OCSI at risk labour markets including key workers, vulnerable industries, Corona Virus Job Retention Scheme and the Self Employment Income Support Scheme.

Health Vulnerability
The health vulnerability data includes Health Deprivation (IMD 2019), access to Healthy Assets (e.g. GPs, Pharmacies) and Hazards (e.g. gambling and Mean NO2 & SO2), Ethnicity, British Red Cross COVID vulnerability index (metrics include clinical vulnerability, adult obesity, over 70s and vulnerability scores) and suicide rates and common mental health issues data from Public Health England.

Change in Vulnerability
Certain data have been aggregated to indicate which Layer Super Output Areas (LSOA) identify economic vulnerability ((e.g. Income support, Job seekers allowance, carers benefits, employment, household income and level 4 education levels) and identifies those most likely to be impacted in a post COVID19 world (those aged 25-34, those self-employed, those employed in retail and hospitality etc).

In addition to the socio-economic and demographic analysis Emu Analytics has carried out some spatial analysis on the width of pavements in order to identify where people in a specific town or city have access to pavements over or under 2 metres wide. This has potentially significant impact in terms of perceptions of safety for people as well as identifying a requirement in areas with high footfall but pavements under 2 metres to commandeers all or part of the road network for pedestrians which in itself creates wider movement issues for other transport types. Below is an example from Exeter where blue areas indicate pavements over 2 meters in width and the red areas are under 2 metres wide.

Sentiment Analysis
In the background at Didobi we always aim to run a couple of projects where we look at new ways to analyse structured and unstructured data. Earlier this year we used Natural Linguistic Processing (NLP) to look at the relationship between landlords by type of asset class and also retailers by analysing the chairman’s commentary from the annual reports. Our interest lay in the variation in sentiment by company over time relative to each other and then the subsequent performance in their share price. Below are some of the outputs and of note is the variance that does exist along with the detailed example of Intu where the sentiment index reflected the share price. Remember of course that a Chairman’s statement has key indicators in it with regards the future perceived future performance of the company relative to the market conditions at the time.





Inclusion of other market data beyond share price (not applicable to all) can also be interesting when you look at the difference in sentiment between retailers and landlords and prime yields.

Tenant Income Risk
More recently a new business called Income Analytics launched that has created a set of unique models and algorithms to measure and forecast income risk at a tenant, building, fund and portfolio level. For the first time large volumes of data have been aggregated, analysed and modelled to create an equivalent bond rating for commercial real estate income. In many ways Income Analytics has created a Google Translate service to enable real estate owners, investors, advisers and lenders to have a common set of data upon which to measure commercial real estate income. This is now more important than ever in the current global economic climate.

Through a data partnership agreement with Dun & Bradstreet, Income Analytics is able to analyse risk on over 330 million companies globally in this way which means a detailed understanding of counter party risk at all levels can be achieved and then rolled up to whatever level of investment vehicle is required. 350 million updates occur daily in the database and so the power of cloud computing can be leveraged to deliver a comprehensive and current view at a point of time as well as these projected out 10 years. In the UK the average lease length is currently 7 years’ so this enables businesses to better understand and model risk.

Recent analysis of companies in certain retail sectors illustrates clearly the change in fortunes and the level of stress being experienced by companies. Understanding the corporate family tree of companies is also a key indicator as to how they might fare or behave when it comes to paying rent. The news has been full of examples of certain retailers not paying rent but understanding the difference between Can Pay Won’t Pay and Can’t Pay Won’t Pay is key. Analysis of such retailers proves interesting when you look at the difference between private equity owned businesses, publicly listed businesses and privately owned businesses. Below is a good example of how the Income Analytics data shows the stark differences in risk of three retailers from virtual parity twelve months ago to polarisation today.

So, as you can see the ability to use data to make informed decisions, to answer questions and solve problems is available. Understanding how to use data and what story the data is telling you is the key to success. All too often people have the answer they want and then fit the data to the answer. Such an approach will result in the failure of the business or organisation that such a service purports to support.
The key stages for success are;
- Know the question or the problem you are trying to solve
- Identify the data that best relates to the issue in question
- Audit the data for accuracy, integrity, currency and comprehensiveness
- Analyse it in multiple ways
- Interpret and visualise the story that the data is telling you
- Apply the data narrative to solving your issue
- Monitor changes in the data to see if it changes the execution of issue resolution
“The goal is to turn data into information and information into insight.” Carly Fiorina, former chief executive officer, Hewlett Packard.
Matthew Hopkinson, 3rd July 2020.
Leave a comment: